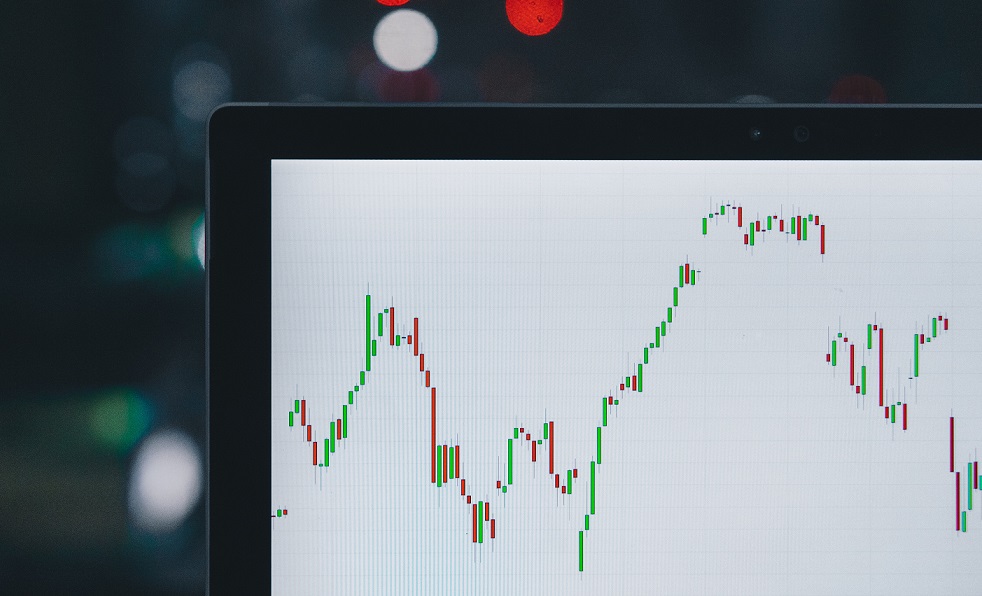
Why are publications given so much importance? Is it because they convey a telling story about the on-going research(s) in the world? Is it because they act as ‘validation’ for a researcher? Or is It because it helps other researchers to use existing literature to develop their own research questions? I believe, all these reasons are true, to some extent or other. If that the case, then should we trust only the researchers who have a greater number of published articles/research papers in their names? If that is the case, then we surely are reading only the articles which were able to answer the research questions and ignoring the ones which could not.
But, why is it even important to read something that could not even answer a simple research question? Should we ignore the studies which are not directly helpful to another research?
I believe, we should not, because it is equally important to read about what works and what does not work in an experimental design. If we do so, then the debate takes a different angle altogether, as we begin giving some importance to the research work which is not very often recognized. But, we see that this is important to consider, as good policy decisions are an outcome of a contextual research question, a well-thought research design, useful quality data, credible methodology, and compelling results. Sadly, not all such research outcomes are known to the world, and the most common reason is that the results are not captivating enough. In simpler words, failed experiments are not often published.
We discuss two problems relating to current phenomena of publication:
- Specification searching – selective reporting of analyses within a particular study;
- Publication bias: It occurs when the outcome of an experiment or research study influences the decision whether to publish or otherwise distribute.
Both problems are deep-rooted, as sometimes researchers try to fudge the data, manipulate the methodologies, or modify the research question to make sure their work gets recognized. Many research ideas die in infancy when the researchers are ‘unable to reject the null hypothesis’, i.e. they are ‘unable to obtain statistically significant’ results. What we miss in the picture is that failing to reject the null hypothesis is not the same as ‘accepting the null hypothesis’, it only means that the study is unable to provide enough evidence for the alternative hypothesis to be true. During such times, one must remember: “ There are lies, damn lies, and statistics”. Statistics and econometrics are mere tools to analyse, and interpret the data, but not the only tools we should we depend on. Practical significance is of equal, rather more important than statistical significance, because statistically significant results might be impractical : example, the outcome variable is ‘ female labour force participation’, and one of the explanatory variable ‘ age squared’ then has a positive coefficient, and is statistically significant at p-value of less than 5 %, which means that as women age, labour force participation increases. This is counter-intuitive (or atleast context specific), and in such a scenario, a researcher has two options : one, keep adding more explanatory variables to get the desired results, or two, stick to the current model, and try to look at the intuitive reasons behind the result (the case of Japan, where demographics are such, that older people participate more in the labourforce). Let’s consider another scenario, in which ‘age squared’ has a positive coefficient, but is statistically insignificant. In this case, the researcher might try to play with different models to arrive at a ‘significant’ coefficient, which is the problem of ‘specification searching’. Such scenarios often happen when researchers want to make their work publishable with ‘compelling’ results. Journals do not find such papers interesting enough to publish, and this why most experiments that fail are neither ever heard of, nor studied.
“I have not failed, I have just found 10,000 ways that won’t work” – Thomas A. Edison. The importance of publishing failed experiments is highly under-rated. There is very little literature on which experiments failed, what methodology did they use, and why did they fail. This literature is imperative to understand what new changes should we look at, and what new strategies should we follow to get the desired results. Plenty of theories about what works in an experiment, but nobody talks about what happens on the field when we work, and why we fail to get the desired results. Since failed experiments are not published, we get to read upon only those ‘wonderful’ experiments, that managed to reject the null hypothesis. All the researchers have a common goal: to portray reality of the world. Why is it then the researchers with concrete reality can reach heights in their career, and not the failed ones? Isn’t it essential to look at the reasons behind the failure, the research design, their methodology, their data quality? It is equally possible that two researchers with same research question, work using two different methodologies, and datasets. It is definitely possible that one of them gets their work published because he could get ‘publishable’ results, but had a not-so-reliable methodology, while the other, had incredible methodology, but poor data quality, due to which the undesired results appeared. When we do this, we miss out on interesting negative findings, which may help us in asking more relevant questions about research.
There are organizations like WHO, TESS which have a dedicated section to publish failed experiments. Recently, a proposal was made in the U.S.A., wherein it was discussed that initially, if an abstract of the research paper of a PhD student is reviewed and accepted, then, the findings will be published, no matter what the outcome of the study is. However, the implementation of this policy is long due, and till then, we are hopeful about minimizing the publication bias and maximizing efforts in optimizing research designs and methodologies, and improved data quality, to ensure unbiased research.
“Whether you fear it or not, true disappointment will come, but with disappointment comes clarity, conviction, and true originality.” – Conan O’ Brien.
Komal is presently working as a research associate at NEERMAN, a research and consulting firm based in Mumbai. Prior to joining NEERMAN, she interned at National Institute of Public Finance and Policy, a think-tank based in New Delhi, focusing on studies that relate to gender budgeting.
She has completed her Masters’ in Economics from Gokhale Institute of Politics and Economics and has majored in Economics from the University of Delhi.