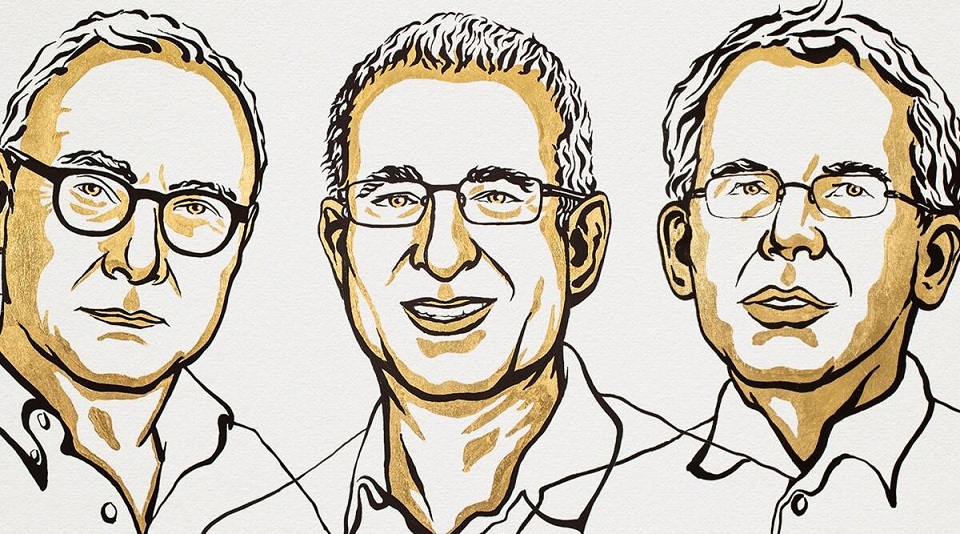
Animals of the Arctic have several adaptations to help them survive in a cold, inhospitable climate. The polar bears, for example, possess white fur that helps them camouflage in the environment because it blends in with the landscape. Additionally, they are kept warm by their blubber/fat to survive the freezing cold Arctic. Polar bears also have pads on their feet, to avoid slippages and accidents on the slippery ice. Now, imagine a counterfactual situation wherein the polar bears were transferred to a hot, sweltering landmass. Will they be able to survive and thrive in that situation? Research-based evidence indicates that in the regions where terrestrial feeding by polar bears has been documented, polar bear body condition and survival rates have severely declined. Polar bears are so adapted to suit a cold environment that their useful characteristics fail to serve as strengths in a completely new environment. Natural Experiments conducted to identify cause-and-effect relationships can often work this way.
The benefits of natural experiments to create innovative research designs, aimed at finding credible evidence to answer important policy questions are well-known. Recently, David Card, Joshua D. Angrist, and Guido W. Imbens received the prestigious Nobel Prize 2021 award for offering new insights on labour markets and showing what conclusions about cause-andeffect can be drawn from natural experiments. In a rough-and-ready sense, a natural experiment stands for real-world situations that are studied for their effects on individuals. They arise when comparable individuals or groups of people are sorted by “nature” into something like a control and treatment group. The key to these observational experiments is that the results are often “serendipitous”, or ones leading to surprising new discoveries. The ability of natural experiments to provide causal estimates for complex topics makes them very popular across many different disciplines. A major benefit of natural experiments lies in the fact that they occur frequently in economies, for example, say due to policy changes in some regions of a country or income thresholds in tax and benefit systems, which mean that some individuals are exposed to an intervention while other, similar, individuals are simply not. This unintended randomness provides researchers with opportunities for uncovering causal relationships in real-life scenarios, minimising issues of bias in sample selection and ensuring high ecological validity. Another advantage of such experiments is a low likelihood of demand characteristics affecting the results, as participants often do not know they are being studied. These experiments are also valuable in situations in which it would be ethically unacceptable to manipulate the independent variable (conducting experiments, like RCTs) for example, conducting studies on impact of stress on the immune system, and mental and physical wellbeing of individuals.
However, there are a few roadblocks that often come in play with natural experiments. The “external validity” of a natural experiment (i.e., extent to which you can generalize the findings of a study to other situations, people, settings, and measures) conducted is often questionable. For example, the Angrist and Imbens analysis has played a key role in Card’s (2001) interpretation of the literature on returns to schooling, and the insights have been used and extended by numerous researchers since. But what the study also highlights is that any single experiment will only provide partial results on a question. To gain a fuller picture, we need more analyses with different instruments or otherwise different individuals affected in other geographies. These issues of external validity are extremely crucial before governments and policymakers jump to formulating policies basis a few localised experiments. Another limitation in natural experiments resides in no control over extraneous variables and the subsequent difficulty for a researcher to replicate the same study in a different area. For example, the administering of a second dose of a particular covid vaccine after the first dose of another in Siddharthnagar district of Uttar Pradesh in May’21 in India after Covid was a natural experiment for information on the efficacy of a vaccine cocktail in comparison with both doses of a single vaccine. But how can we replicate variables like – the efficiency and control of government administration and community-level awareness outreach, or the public health infrastructure in the state or the general health & life expectancy of its denizens, in other potential future experiments for comparison or addition to existing body of knowledge?
A few internal and external policy recommendations can help improve efficiency and reliability of natural experiments. Internally, to tackle the major limitation of inferring causation from natural experiments due to the presence of unmeasured confounding, few econometric methods are often implemented by economists. One class of methods designed to control confounding and measurement error is based on instrumental variables (IV). IV analyses depend on the assumption that subjects were effectively randomized, even if the randomization was accidental (in the case of an administrative policy change or exposure to a natural disaster). Externally, the results and discoveries of natural experiments is limited in scope and coverage and thus, should not be quickly presumed to drive policy in a particular area. Good intentions do not necessarily translate to good policies. Therefore, further experiments, affiliated studies and historical recommendations must be leveraged before adopting a policy. The lack of random assignment means multiple threats to causal inference, including attrition, history, testing etc. may influence observed study outcomes. For this reason, natural experiments will never unequivocally determine causation in particular situations. Nevertheless, if used with care, they can provide additional data that may be useful for a research question and that may not be obtainable in any other way.
These are author’s personal views. Himanshi Goel is currently working as a Independent Consultant advising multilateral institutions. Previously, she has worked with analytics consulting organizations like Experian, advising banks, NBFCs, and FinTechs on credit risk solutions. She is an alum of the Delhi School of Economics.